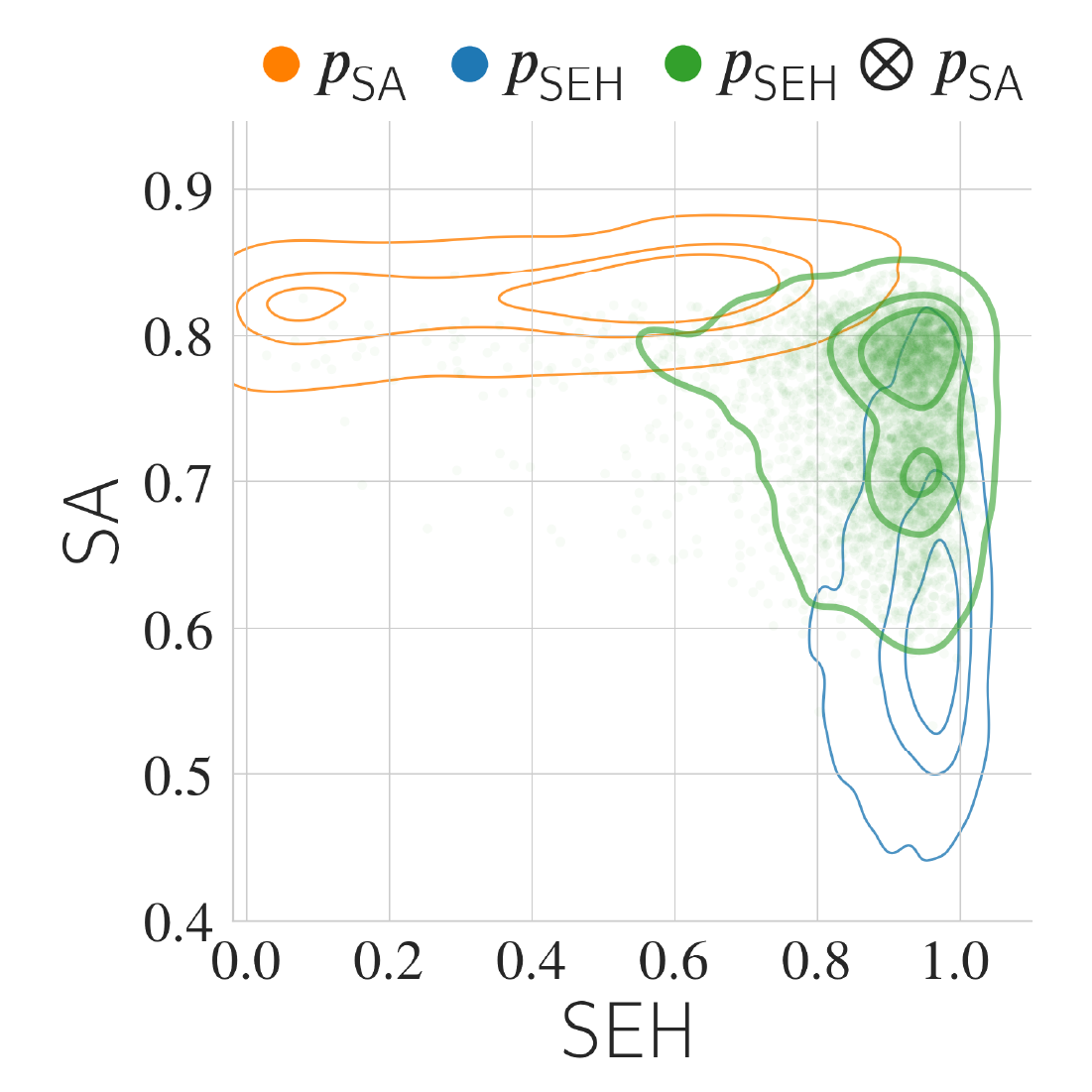
Compositional Sculpting of Iterative Generative Processes
, Sebastiaan De Peuter, Ge Yang, Vikas Garg, Samuel Kaski, and Tommi Jaakkola
NeurIPS 2023: Neural Information Processing Systems
Timur Garipov
email: <firstname>@csail.mit.edu
I am a PhD student in Computer Science at MIT EECS & MIT CSAIL, where I am fortunate to be advised by professor Tommi Jaakkola. My research is focused on probabilistic machine learning and deep learning. I am interested in generative models, empirical approaches to understanding training, robustness, and generalization of deep neural networks.
I received a BSc and MSc in Applied Mathematics and Computer Science from the Lomonosov Moscow State University, where I worked in Bayesian Methods Research Group supervised by Dmitry Vetrov.
In the summer of 2021, I completed a research internship at Google Brain, working with Chiyuan Zhang. In the summer of 2023, I completed an internship at Cruise AI Research team supervised by David Hayden.
Google Scholar / Twitter / Github
News: I defended my PhD thesis "Guiding Deep Probabilistic Models".
Compositional Sculpting of Iterative Generative Processes
, Sebastiaan De Peuter, Ge Yang, Vikas Garg, Samuel Kaski, and Tommi Jaakkola
NeurIPS 2023: Neural Information Processing Systems
Adversarial Support Alignment
Shangyuan Tong, , Yang Zhang, Shiyu Chang, and Tommi Jaakkola
ICLR 2022: International Conference on Learning Representations
Spotlight Presentation
Subspace inference for Bayesian deep learning
Pavel Izmailov, Wesley J. Maddox, Polina Kirichenko, , Dmitry Vetrov, and Andrew Gordon Wilson
UAI 2019: Uncertainty in Artificial Intelligence
A Simple Baseline for Bayesian Uncertainty in Deep Learning
Wesley J. Maddox, , Pavel Izmailov, Dmitry Vetrov, and Andrew Gordon Wilson
NeurIPS 2019: Neural Information Processing Systems
Averaging Weights Leads to Wider Optima and Better Generalization
Pavel Izmailov, Dmitrii Podoprikhin, , Dmitry Vetrov, and Andrew Gordon Wilson
UAI 2018: Uncertainty in Artificial Intelligence
Oral Presentation
Loss Surfaces, Mode Connectivity, and Fast Ensembling of DNNs
, Pavel Izmailov, Dmitrii Podoprikhin, Dmitry Vetrov, and Andrew Gordon Wilson
NeurIPS 2018: Neural Information Processing Systems
Spotlight Presentation
The Benefits of Pairwise Discriminators for Adversarial Training
Shangyuan Tong, , and Tommi Jaakkola
Preprint 2020
Subspace Inference for Bayesian Deep Learning
Pavel Izmailov, Wesley J. Maddox, Polina Kirichenko, , Dmitry Vetrov, and Andrew Gordon Wilson
ICML Workshop 2019: Uncertainty & Robustness in Deep Learning
Oral Presentation
Ultimate tensorization: compressing convolutional and FC layers alike
, Dmitrii Podoprikhin, Alexander Novikov, and Dmitry Vetrov
NIPS Workshop 2016
Contact-Aware Lyapunov Controller Design via Alternating Optimization
Richard Li and
Class project MIT 6.832 (now 6.8210): Underactuated Robotics (Spring 2022)
Instructor: Russ Tedrake
Robotic Arm Weightlifting via Trajectory Optimization
Class project MIT 6.843 (now 6.4212): Robotic Manipulation (Fall 2021)
Instructor: Russ Tedrake
Implementation of Algorithms for Construction of Voronoi Diagram
Class project MIT 6.850 (now 6.5320): Geometric Computing (Spring 2020)
Instructor: Piotr Indyk
Fast Uncertainty Estimates and Bayesian Model Averaging of DNNs
Wesley J. Maddox, , Pavel Izmailov, and Andrew Gordon Wilson
UAI Workshop 2018: Uncertainty in Deep Learning
Oral Presentation
Improving Stability in Deep Reinforcement Learning with Weight Averaging
Evgenii Nikishin, Pavel Izmailov, Ben Athiwaratkun, Dmitrii Podoprikhin, , Pavel Shvechikov, Dmitry Vetrov, and Andrew Gordon Wilson
UAI Workshop 2018: Uncertainty in Deep Learning
Bayesian incremental learning for deep neural networks
Max Kochurov, , Dmitry Podoprikhin, Dmitry Molchanov, Arsenii Ashukha, and Dmitry Vetrov
ICLR Workshop 2018